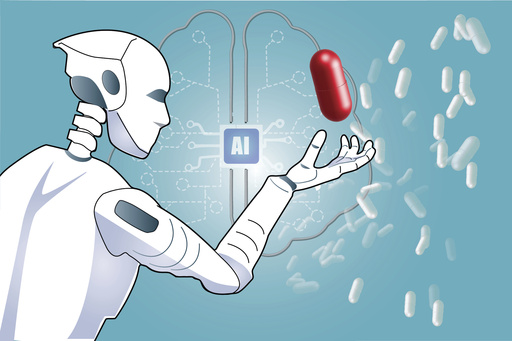
WASHINGTON — The landscape of business operations is rapidly evolving due to artificial intelligence, with innovations aiding programmers in code creation and chatbots enhancing customer service.
However, the pharmaceutical sector continues to seek effective AI applications to address one of its most pressing challenges: streamlining the drug development process.
Despite extensive investments amounting to billions of dollars into research initiatives, new pharmaceuticals generally require over a decade to bring to market.
Since its inception in 2018, Insitro has emerged as a key player among a growing number of AI-driven companies dedicated to expediting the drug discovery process. By utilizing machine learning to sift through vast datasets comprising chemical and biological markers, Insitro aims to innovate drug development.
Headquartered in South San Francisco, the company has established partnerships with prominent pharmaceutical firms such as Eli Lilly and Bristol Myers Squibb, focusing on creating treatments for metabolic diseases, neurological issues, and degenerative disorders.
In a recent discussion, Insitro’s CEO and founder, Daphne Koller, shared insights about the role of AI in overcoming the hurdles of drug discovery. The following conversation has been condensed for brevity.
Q: Why is drug development so challenging?
A: Drug discovery is complex mainly due to our limited understanding of biological systems. The most notable breakthroughs in recent years have stemmed from times when we comprehended these systems well enough to design effective interventions.
At Insitro, we focus on dissecting the intricate nature of heterogeneous diseases and identifying potential intervention tactics. These could be applicable to specific patient subsets rather than the whole population. By honing in on the right therapeutic approaches tailored to distinct patient groups, we hope to address some of the industry’s challenges effectively.
Q: Major pharmaceutical firms like Eli Lilly employ a multitude of medical experts. How does your technology enhance their capabilities?
A: The rise of AI coincides with a significant evolution in what I refer to as quantitative biology, which involves the precise measurement of biological systems. Breakthroughs in technology now allow for detailed assessments of proteins and cells.
However, when humans are presented with overwhelming data, it can become challenging for them to discern subtle variations within. Leave it to individuals, and they may overlook critical insights due to innate limitations in perceiving complexities within multifunctional systems. This renders a simplistic interpretation that may obstruct our understanding of patient differentiations and the potential impact of various interventions.
Q: What motivated your interest in this field?
A: With a PhD in computer science, my venture into machine learning and biomedical applications sparked around 1998 or 1999. In earlier stages, the machine learning problems presented were somewhat mundane—classifying emails as spam or not, for instance—failed to ignite my curiosity.
I pursued a path with more complexity. Although my initial aim wasn’t to delve into biology, the depth of questions in this area drew in my interest, and I found myself increasingly captivated by biological concepts.
Q: Insitro brings together computer scientists and medical professionals. Did you face challenges in merging these two cultures?
A: Overcoming such cultural barriers has been one of our organization’s most significant accomplishments. Despite having diverse expertise, individuals from either domain might struggle to communicate effectively.
Engineers typically seek to identify definitive, consistent patterns that facilitate predictions across a broad population. Contrarily, life scientists aim to uncover exceptions, as these anomalies may lead to groundbreaking discoveries. To bridge this gap, we have implemented various cultural and organizational strategies that encourage open, respectful, and constructive collaboration between both groups.