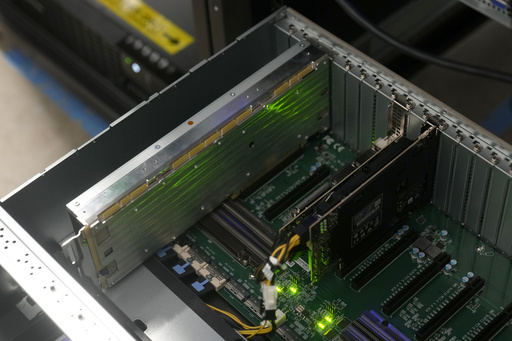
SANTA CLARA, Calif. — The development of current artificial intelligence (AI) chatbots has heavily depended on specialized computer chips developed by Nvidia, which has become synonymous with the AI innovation surge. However, the very characteristics that make Nvidia’s graphics processor units (GPUs) so effective in building powerful AI systems present challenges when it comes to their operational efficiency in everyday applications of AI.
This has created openings for competitors in the AI chip market who believe they can rival Nvidia by providing AI inference chips. These chips are designed to be better suited for the practical application of AI technologies and are aimed specifically at helping reduce the substantial computing costs associated with generative AI. Jacob Feldgoise, an expert at Georgetown University’s Center for Security and Emerging Technology, noted, “These companies see an opportunity for specialized hardware that caters to the increasing demand for inferences as the adoption of AI models grows.”
AI inference refers to the computational processes that occur once an AI system has been fully trained. Initially, AI chatbots undergo a significant training phase where they learn from large datasets. GPUs excel at this function because they can perform numerous calculations simultaneously across interconnected devices. Nonetheless, when a trained generative AI model is called upon to generate text or create an image after the training phase, it requires a different approach involving inference. This involves the AI utilizing its learned data to process new inputs and generate responses. While GPUs can perform this task, they can be overkill for the lighter demands of inference work, which involves less computational power.
As a result, startups like Cerebras, Groq, and d-Matrix are emerging alongside traditional chip makers like AMD and Intel, all focusing on marketing chips better suited for inference tasks. This comes as Nvidia continues to prioritize the high demand for its advanced hardware from major tech firms.
D-Matrix, a company founded in 2019, is set to unveil its first product this week. CEO Sid Sheth shared that entering the AI chip sector later than many competitors posed challenges, particularly as the pandemic shifted the tech industry’s focus toward software solutions for remote work. However, he is optimistic about the burgeoning market for AI inference chips, likening it to the way humans apply knowledge gained from education throughout their lives.
The company’s flagship product, named Corsair, incorporates two chips comprising four chiplets each, manufactured by Taiwan Semiconductor Manufacturing Company — also the supplier for many Nvidia chips. The design process occurs in Santa Clara, with assembly in Taiwan and a comprehensive testing phase back in California that can extend to six months per product.
In the current landscape, tech giants like Amazon, Google, Meta, and Microsoft are rapidly acquiring GPUs to fuel their AI ambitions. Conversely, manufacturers of AI inference chips aim to cater to a wider audience, including Fortune 500 companies that wish to leverage generative AI without needing to invest heavily in the necessary infrastructure. Sheth anticipates strong demand particularly in the realm of AI video content creation.
Analysts have observed that, when it comes to AI inference, the focus is squarely on the speed of response time from chatbots rather than the intensive calculations associated with training chips. An emerging wave of companies is focusing on developing AI hardware capable of executing inference tasks within smaller-scale systems such as personal computers, laptops, and mobile phones.
This shift towards more efficient chip design is significant as it has the potential to drastically lower operational costs for businesses utilizing AI, while also addressing broader environmental and energy concerns. Sheth emphasizes the urgent question surrounding the sustainability of the AI sector: “Are we going to jeopardize the planet in our pursuit of what people term artificial general intelligence (AGI)?”
While speculation on the timeline for achieving artificial general intelligence varies widely, Sheth points out that only a few major tech companies are actively pursuing this goal. “What about the rest?” he questioned. “They can’t all go down the same path.” Many emerging companies do not wish to engage with the enormous AI models due to their cost and energy consumption.
Sheth concludes that the potential of inference as a sector within AI has not been fully recognized, despite ongoing headlines focusing on training models. “I’m not sure people truly understand that inference is going to present a significantly larger opportunity than training. It’s the focus on training that continues to dominate the narrative.”